Is Autopilot An Ai
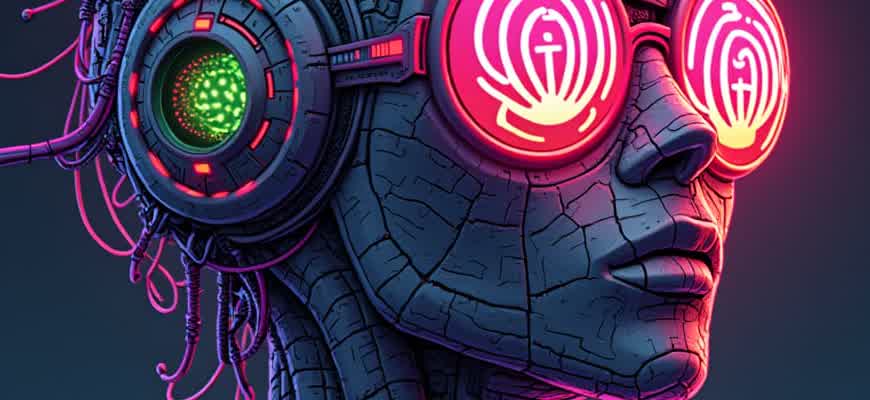
The concept of "autopilot" in the cryptocurrency market has sparked debate about whether it qualifies as true Artificial Intelligence (AI). While often marketed as AI-driven, many autopilot systems are not fully autonomous or capable of independent decision-making. Rather, they are pre-programmed algorithms designed to execute specific tasks based on predefined criteria.
To determine if an autopilot system falls under the umbrella of AI, we need to examine its functionality and decision-making capabilities. The key differentiators between a standard automated system and AI are learning and adaptability. Here’s a comparison:
- Autopilot Systems: Perform tasks according to programmed rules and data inputs.
- AI Systems: Utilize machine learning to adapt, learn from past decisions, and make more informed choices over time.
Key Features of Autopilot vs AI:
Feature | Autopilot | AI |
---|---|---|
Adaptability | Limited | Dynamic |
Learning Capability | None | Yes |
Decision-Making | Pre-programmed | Based on data analysis |
"An autopilot system in crypto may seem intelligent, but its behavior is typically reactive, not proactive. True AI would involve the system improving its strategies and predictions based on real-time market changes."
Exploring the Role of Autopilot Systems in the World of Cryptocurrency
In the fast-paced world of cryptocurrency trading, automation tools are becoming essential for managing investments and maximizing profits. One of the most common forms of automation is known as "Autopilot," a system that runs on algorithms to execute trades or handle other financial decisions without human intervention. But is Autopilot truly an example of Artificial Intelligence (AI), or does it merely mimic some of its functions? Understanding the core mechanics of these systems can shed light on their capabilities and limitations within the context of digital asset management.
Autopilot systems in cryptocurrency often rely on predefined rules or machine learning models to make decisions based on real-time market data. While these systems can seem intelligent, they aren't necessarily "smart" in the same way as AI-driven tools. The key difference lies in how decisions are made: traditional autopilot systems follow specific, hard-coded instructions, whereas AI-based tools are designed to learn and adapt from data over time, improving their performance autonomously.
Capabilities of Autopilot Systems in Cryptocurrency
- Automated Trading: Autopilot can execute trades based on specific market conditions, such as price fluctuations or market trends, without the need for continuous human input.
- Risk Management: These systems often incorporate stop-loss and take-profit mechanisms to manage risk, ensuring that potential losses are minimized in volatile markets.
- Backtesting: Autopilot tools can analyze historical market data to simulate trading strategies, optimizing them for future use.
Key Differences Between Autopilot and AI
- Rule-based vs. Learning: Autopilot operates on a set of predefined rules, while AI can adapt and learn from past data to improve its decision-making abilities.
- Complexity: AI systems are typically more complex, processing large amounts of data to identify patterns, whereas autopilot systems work within a more limited set of instructions.
- Adaptability: AI can dynamically adjust its approach based on changing market conditions, whereas autopilot systems may require manual adjustments.
Important: While autopilot tools in crypto trading provide convenience, they do not offer the same level of adaptability or learning capabilities as true AI systems. Therefore, while they can automate certain tasks, they do not possess the cognitive flexibility of AI-driven platforms.
Comparison Table: Autopilot vs. AI in Cryptocurrency
Feature | Autopilot | AI |
---|---|---|
Decision-making | Based on predefined rules | Based on data analysis and learning |
Adaptability | Limited, requires manual updates | High, adapts to market changes |
Complexity | Simple algorithms | Advanced models with pattern recognition |
What is the Technology Behind Autopilot Systems?
Autopilot systems rely on a combination of advanced sensors, software, and machine learning algorithms to navigate vehicles with minimal human intervention. In the context of autonomous driving, these technologies have become highly integrated into various systems, from cryptocurrency-enabled vehicles to automated drones. The aim is to provide a seamless experience by allowing the vehicle to "understand" its surroundings and make decisions based on real-time data.
In the world of cryptocurrencies, similar technologies are being applied to manage autonomous transactions and asset management. Blockchain technology and smart contracts are used to automate processes that would otherwise require human oversight, much like the autopilot in vehicles. This parallel between cryptocurrency and autopilot systems highlights the role of automation and AI in improving efficiency and reducing the need for manual input.
Core Components of Autopilot Systems
- Sensors - Devices like cameras, LIDAR, radar, and ultrasonic sensors are key to collecting data about the vehicle's surroundings.
- Control Algorithms - Software that processes input from sensors and determines the appropriate response to changes in the environment.
- Machine Learning - AI models are continuously trained to improve the system’s decision-making by analyzing vast amounts of data.
Autopilot System Workflow
- Data Collection: Sensors scan the environment to gather real-time data about obstacles, road conditions, and other variables.
- Processing: Control algorithms analyze the sensor data to understand the vehicle's surroundings and make decisions.
- Action: The system takes action by adjusting speed, steering, and braking to ensure safe navigation.
Autopilot systems represent a convergence of advanced hardware and software, enabling vehicles to navigate complex environments with minimal human interaction, just like automated processes in cryptocurrency transactions.
Technology Behind Cryptocurrency Autopilot
Technology | Purpose |
---|---|
Blockchain | Ensures transparency and security by recording every transaction on a distributed ledger. |
Smart Contracts | Automates transactions and contract execution without the need for intermediaries. |
Machine Learning | Analyzes trends in the cryptocurrency market to predict price fluctuations and automate trading decisions. |
Key Differences Between Autopilot and Full AI Systems in Crypto
In the world of cryptocurrency, both autopilot systems and full AI systems play critical roles, though they operate on different levels. While autopilot systems are often designed for specific tasks and follow pre-set algorithms, full AI systems are capable of learning, adapting, and making complex decisions in dynamic environments, such as the volatile crypto market. Understanding these distinctions is essential for investors and developers working within the space.
Autopilot systems, in the context of cryptocurrency, typically refer to automated trading bots or platforms that execute trades based on predefined rules. These systems do not adapt beyond their programmed constraints, whereas full AI systems, such as machine learning algorithms, analyze vast amounts of data, adjust strategies in real-time, and predict market trends based on evolving patterns.
Key Differences
- Level of Decision-Making:
- Autopilot: Executes tasks based on pre-programmed rules without learning from the environment.
- Full AI: Learns from real-time data and adapts its strategies based on new insights.
- Complexity:
- Autopilot: Simpler and more predictable with limited scope of action.
- Full AI: Highly sophisticated, capable of making complex predictions and handling unexpected market changes.
- Market Adaptation:
- Autopilot: Works with fixed parameters and may struggle in highly volatile markets like crypto.
- Full AI: Continuously adapts, learning from real-time market conditions and improving its performance over time.
Full AI systems can process data in ways that autopilot systems simply cannot, especially in markets as unpredictable as cryptocurrency. Autopilot relies on static rules, while AI can generate insights that evolve with market trends.
Comparison Table
Feature | Autopilot | Full AI |
---|---|---|
Learning Ability | No learning, static programming | Continuous learning, adapts to new data |
Market Strategy | Pre-defined, rigid | Dynamic, can evolve strategies |
Execution | Follows simple instructions | Can handle complex decision-making |
Risk Management | Basic, relies on preset parameters | Advanced, analyzes multiple risk factors |
How Autopilot Makes Real-Time Decisions in Cryptocurrency Markets
In cryptocurrency trading, real-time decision-making is essential for maintaining profitability and minimizing risk. Autopilot systems used in this context often leverage advanced algorithms to process data, predict market movements, and execute trades autonomously. These systems are designed to operate based on a set of predefined rules and machine learning models, ensuring that each decision is made swiftly and accurately without human intervention.
Autopilot mechanisms typically rely on real-time data feeds, including price changes, trading volume, and historical market trends. By processing this vast amount of data, these systems can analyze patterns, detect anomalies, and make informed decisions on buying or selling assets. The goal is to achieve optimal trading outcomes while minimizing the risk of significant losses, even during volatile market conditions.
Key Components of Autopilot Decision-Making in Crypto Trading
- Market Sentiment Analysis: Real-time sentiment analysis tools help determine the general market mood by processing news articles, social media trends, and public sentiment on various platforms.
- Technical Indicators: Autopilot systems utilize various technical indicators, such as Moving Averages, Relative Strength Index (RSI), and Bollinger Bands, to evaluate the market conditions.
- Machine Learning Models: Advanced machine learning algorithms adapt and improve over time, enabling the system to predict future market movements with increasing accuracy.
How Autopilot Systems Make Quick Decisions
- Data Acquisition: Autopilot systems continuously collect real-time market data, including price, volume, and external market news.
- Data Processing: The system processes this data using various algorithms, including statistical models and deep learning to identify trading opportunities.
- Trade Execution: Once a trading signal is identified, the system executes buy or sell orders automatically based on the analysis performed.
"Autopilot systems in crypto trading make decisions in real-time by analyzing large volumes of data and applying predictive models, enabling them to act faster than human traders."
Real-Time Performance of Autopilot in Crypto Trading
Indicator | Impact on Decision |
---|---|
Price Fluctuations | Rapidly influences buying or selling actions. |
Market Volatility | Higher volatility increases risk, requiring more frequent decision-making. |
News and Social Media | Sentiment analysis determines market direction based on public perception. |
Is Autopilot Limited to Autonomous Vehicles?
The term "autopilot" often conjures images of self-driving cars, but its application goes far beyond just vehicles. As technology continues to evolve, autopilot systems are being integrated into various industries, including finance and cryptocurrency. In the world of digital assets, autopilot functionality is increasingly used in automated trading platforms, allowing users to execute trades based on predefined strategies without manual intervention. These systems aim to reduce human error and improve trading efficiency, much like autonomous vehicles aim to improve road safety by reducing the reliance on human drivers.
Autopilot, in its broader form, is not confined to a singular use case. While autonomous vehicles rely on complex algorithms to navigate roads, autopilot in cryptocurrency trading involves sophisticated machine learning algorithms that monitor market trends, execute trades, and manage portfolios. Below, we explore how these systems function and how they might be integrated into various fields beyond transportation.
Autopilot in Crypto Trading
Autopilot systems in the crypto space are increasingly being leveraged for automated trading strategies. These systems can analyze vast amounts of market data, make quick decisions, and execute trades in real time. The potential for reducing emotional decision-making and increasing the speed of execution has made autopilot systems attractive to crypto traders.
"Automated trading removes the need for constant monitoring, enabling crypto traders to respond faster than ever before."
- Algorithmic trading systems scan market data for trends.
- They use pre-programmed strategies to execute trades automatically.
- Autopilot trading helps mitigate human error and emotional bias in decision-making.
Applications Beyond Vehicles
While most people associate autopilot with autonomous vehicles, these systems have far-reaching applications in various industries, including finance, healthcare, and even content creation. In the financial world, for example, autopilot algorithms are used for everything from portfolio management to high-frequency trading. These systems rely on artificial intelligence and machine learning to adapt to market changes and make decisions without human input.
- Autopilot can be applied to portfolio management in crypto.
- AI-driven bots monitor the market 24/7, executing trades based on pre-determined strategies.
- Autopilot systems provide a faster response time to sudden market shifts compared to manual trading.
Future of Autopilot in the Crypto World
As the technology behind autopilot systems continues to evolve, the scope of their use in crypto trading is expected to expand. Blockchain networks and decentralized finance (DeFi) platforms may integrate more sophisticated autopilot features to create fully autonomous trading environments. This could further reduce the need for human intervention and lead to more efficient market behaviors.
Advantage | Disadvantage |
---|---|
Speed: Autopilot systems make decisions much faster than humans. | Risk: Automated systems can make mistakes or act on outdated data. |
24/7 Operation: Autopilot systems operate around the clock, maximizing trading opportunities. | Lack of Adaptability: Systems may struggle with unexpected market events or black swan scenarios. |
How Autopilot Adapts Through User Input and Market Conditions
Autopilot systems in cryptocurrency trading utilize data from both user actions and external market fluctuations to optimize their strategies. By observing a user's historical trades, preferences, and risk appetite, the system adjusts its algorithms to predict more successful trading outcomes. This personalization helps create an adaptive trading assistant that learns and evolves over time, offering increasingly relevant suggestions and strategies for trading digital assets.
Environmental factors such as market volatility, news, and global events also influence the decisions made by the Autopilot system. By analyzing real-time market data alongside historical trends, these systems adjust their strategies to reflect sudden price changes, shifts in trading volume, and external events that impact cryptocurrency values. This combination of user input and market data forms a dynamic feedback loop that allows Autopilot systems to refine their actions continuously.
Learning Process of Autopilot Systems
- Interaction with User Data: The system tracks user actions, such as trading frequency, asset selection, and set parameters like stop-loss. This information allows it to adjust its strategy for better alignment with user preferences.
- Market Data Analysis: Autopilot systems analyze real-time price changes, volume shifts, and broader market trends, adapting their strategy accordingly.
- Continuous Adaptation: The system evolves by processing new feedback, such as adjusted trade parameters or market responses, to refine future predictions and recommendations.
Key Elements That Influence Learning
- Price Fluctuations: Cryptocurrencies can experience rapid price swings, requiring the system to adjust quickly to these changes.
- Risk Preferences: The system adapts based on user-set risk profiles, ensuring that the strategies align with the user’s risk tolerance.
- External Influences: News events, regulatory changes, and macroeconomic factors play a significant role in shaping trading strategies within the system.
Important: The adaptability of Autopilot systems is driven by both user-specific data and real-time market conditions, creating a continuously improving and reactive trading strategy.
Data and Strategy Adjustments
Data Type | Effect on Strategy |
---|---|
User Behavior | Informs personalized decision-making, fine-tuning trade recommendations to better suit individual preferences. |
Market Trends | Adapts to shifts in price trends, volume changes, and the overall market direction, ensuring that the strategy remains relevant. |
Global Events | Integrates information from news and regulatory changes to mitigate risks and take advantage of emerging opportunities. |
AI’s Contribution to the Development of Autonomous Systems
In the realm of autonomous systems, AI plays a crucial role in enhancing decision-making processes and ensuring the safety and reliability of autopilot technology. With the integration of advanced algorithms, machine learning, and neural networks, AI systems are able to process large volumes of data and adapt to changing environments in real time. This results in more accurate navigation, predictive analytics, and the ability to make instantaneous adjustments based on environmental factors, such as weather or road conditions.
As the technology evolves, AI’s contribution extends beyond basic automation. It now involves more sophisticated tasks such as pattern recognition, anomaly detection, and predictive maintenance. These capabilities significantly reduce the risk of human error and ensure that autonomous systems operate efficiently. The evolution of autopilot systems relies heavily on the continual advancements in AI technologies, creating smarter, more adaptive systems that are becoming increasingly reliable in complex, dynamic environments.
Key Elements of AI in Autopilot Systems
- Data Processing: AI systems continuously analyze vast amounts of sensor data to identify patterns, allowing autopilot systems to understand their surroundings and make informed decisions.
- Real-time Adaptation: Through machine learning, autopilot systems can adjust their behavior based on real-time data, optimizing performance and safety in dynamic environments.
- Decision-making Algorithms: AI algorithms help the system make quick decisions in critical situations, like obstacle avoidance or emergency braking, by evaluating numerous variables at once.
AI and Machine Learning Techniques in Autopilot Development
- Supervised Learning: Training models on labeled data to improve recognition accuracy for objects, hazards, and road signs.
- Reinforcement Learning: AI systems learn from trial and error, improving performance in complex tasks such as navigation or route optimization.
- Deep Learning: Deep neural networks analyze high-dimensional data for more advanced recognition, enabling the autopilot to make decisions based on intricate patterns.
AI’s continual evolution ensures that autopilot systems become more intuitive, accurate, and safe, with the potential to revolutionize industries beyond transportation, including aerospace and marine navigation.
Future Implications of AI in Autopilot Systems
Technology | Impact |
---|---|
AI Integration | Enhanced adaptability, predictive capabilities, and real-time decision-making in autonomous systems. |
Data Analytics | Improved efficiency through continuous learning and data-driven decision-making processes. |
Advanced Machine Learning | Increased safety and reduced human error through continuous self-improvement of autonomous systems. |
Why Is Autopilot Not Considered Fully Autonomous Yet?
The concept of autonomy in systems like autopilot has been a subject of debate for some time. In the cryptocurrency sector, where advanced algorithms and AI are used to handle transactions and investments, parallels can be drawn to the limitations seen in autopilot systems. Although these systems can automate tasks, they still rely heavily on human oversight and intervention. The same principle applies to AI systems managing cryptocurrency transactions, where certain levels of autonomy are present but complete independence is not achievable yet.
In the context of autopilot systems, complete autonomy would imply that a vehicle or system can operate entirely without human involvement. However, in both the automotive and crypto sectors, true autonomy remains elusive. Below are the key factors that prevent autopilot systems from being fully autonomous.
Key Limitations of Autopilot Systems
- Complex Decision-Making: Autopilot systems are not capable of making fully independent decisions, particularly when faced with unforeseen scenarios or complex variables. For example, in cryptocurrency trading, AI can predict market trends but still requires human judgment for risk assessment.
- Dependence on Data Quality: Both autopilot systems and crypto algorithms depend on the accuracy of input data. Poor data quality can lead to errors, causing the system to malfunction or make inaccurate predictions.
- Legal and Ethical Issues: Just as the law limits how much autonomy a self-driving car can have, cryptocurrency regulations and ethical concerns about AI decision-making are limiting the full implementation of autonomous systems.
Challenges in Achieving Full Autonomy
- Unpredictability of Human Behavior: Autopilot systems cannot fully predict or react to human actions, which is crucial in both traffic situations and crypto trading. The unpredictability of market behavior makes it difficult for AI to make decisions without human input.
- Security Concerns: In both domains, AI systems need to be safeguarded from cyber threats. A fully autonomous autopilot or crypto system might be vulnerable to hacking, making continuous human monitoring necessary.
"Autonomy in systems like autopilot isn't just about technology; it also requires overcoming deep ethical, legal, and practical challenges that still demand human oversight."
Factor | Impact on Autonomy |
---|---|
Data Accuracy | Vital for making correct decisions; unreliable data hampers autonomy. |
Market Uncertainty | In cryptocurrency, human input is needed to handle market volatility and anomalies. |
Legal Restrictions | Regulations prevent full autonomy in both vehicle and financial systems. |