Automl Business
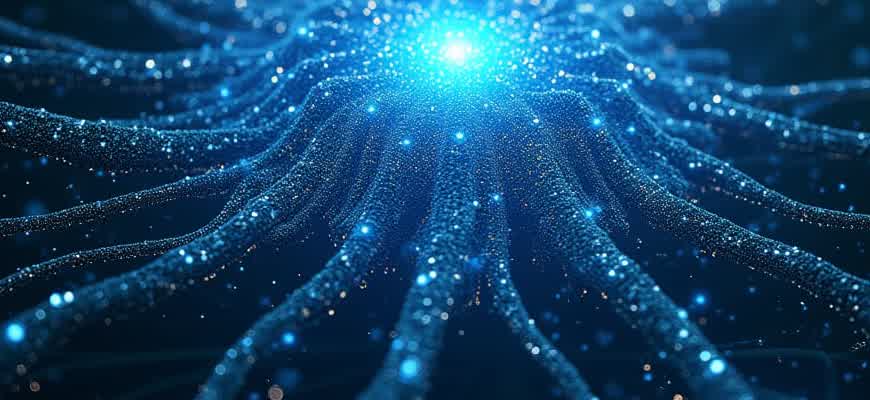
In recent years, the convergence of blockchain technology and artificial intelligence has opened new frontiers in business innovation. The integration of Automated Machine Learning (AutoML) tools has proven to be a game-changer for companies operating in the crypto space. AutoML platforms enable businesses to automate the process of building machine learning models, enhancing decision-making capabilities and operational efficiency in blockchain-related industries.
Key Benefits of AutoML for Crypto Businesses:
- Streamlined data analysis and model selection.
- Reduction in manual intervention, speeding up development cycles.
- Increased scalability in predictive modeling, improving transaction validation and fraud detection.
Applications of AutoML in Crypto
- Predictive analytics for cryptocurrency price trends.
- Fraud detection in decentralized finance (DeFi) platforms.
- Smart contract optimization using data-driven insights.
“The use of AutoML in blockchain companies significantly reduces the barriers to machine learning, allowing firms to focus on their core business operations while automating complex predictive tasks.”
Market Insights
Industry | Growth Rate (2024-2028) | Market Size (2028) |
---|---|---|
Blockchain Solutions | 15% | $45 billion |
AutoML Software | 20% | $10 billion |
How Automl Tools Optimize Data Analysis for SMEs in the Crypto Industry
In the rapidly evolving cryptocurrency market, small and medium enterprises (SMEs) often struggle to make data-driven decisions due to the complexity and volume of available data. Automl tools offer a solution by automating much of the data analysis process, enabling SMEs to quickly extract actionable insights without requiring deep expertise in data science. These tools can process large datasets in real-time, offering faster insights into market trends, investment opportunities, and customer behavior.
Automl systems empower SMEs by reducing the need for specialized data science knowledge, while providing accurate, tailored predictions. This makes them especially beneficial in the fast-paced cryptocurrency landscape, where decisions must be made quickly to stay competitive. By automating the data processing pipeline, Automl platforms allow smaller businesses to leverage advanced analytics without the overhead of hiring dedicated teams of data scientists.
Key Benefits of Automl Tools for Crypto SMEs
- Speed: Automl platforms can analyze vast amounts of cryptocurrency data in real-time, providing timely insights for trading and investment decisions.
- Cost-efficiency: Reduces the need for expensive, in-house data science teams.
- Scalability: As data grows, Automl tools scale accordingly, ensuring continuous performance without additional resources.
Typical Automl Applications in Crypto Analysis
- Price Prediction: Automl tools can forecast price trends by analyzing historical and real-time market data, improving trading strategies.
- Fraud Detection: Automated models can identify unusual patterns and flag potential fraud attempts, helping SMEs mitigate risks.
- Sentiment Analysis: Automl tools can gauge market sentiment from social media, news articles, and forum discussions, providing SMEs with a clearer view of market mood.
By leveraging Automl, crypto SMEs can unlock powerful predictive capabilities that were previously available only to larger enterprises with extensive resources.
Example: Automl in Action for Cryptocurrency Analysis
Tool | Use Case | Benefit |
---|---|---|
CryptoAI | Price Prediction | Improved trading decisions based on real-time data |
BlockGuard | Fraud Detection | Reduced risk of fraudulent transactions |
SentimentBot | Market Sentiment Analysis | Enhanced understanding of public sentiment, improving market positioning |
Optimizing E-commerce with Automated Cryptocurrency-based Personalization Solutions
In the rapidly evolving e-commerce landscape, implementing machine learning solutions is crucial for creating personalized shopping experiences. With the growing integration of cryptocurrencies, businesses have a unique opportunity to tailor user experiences based on transaction behavior and blockchain interactions. Automated Machine Learning (AutoML) is transforming how e-commerce platforms manage and utilize customer data, especially within the cryptocurrency space. By leveraging AutoML, businesses can optimize user profiles and make real-time recommendations based on sophisticated data analysis, which improves conversion rates and customer loyalty.
Crypto-based payment systems introduce additional layers of complexity when it comes to personalization. Transactions through blockchain are transparent, traceable, and decentralized, which provides an opportunity for more secure and personalized experiences for customers. Machine learning algorithms, combined with blockchain data, can be harnessed to build dynamic customer models that reflect not only traditional purchasing behaviors but also wallet transactions, token holdings, and participation in decentralized finance (DeFi) platforms. This allows for a more nuanced approach to delivering personalized product recommendations and offers.
Key Benefits of Integrating AutoML for Crypto-Enabled E-commerce
- Real-Time Personalization: AutoML models can continuously adapt to new data, providing hyper-personalized experiences based on user transactions and preferences.
- Enhanced Data Security: Blockchain’s transparent nature combined with machine learning ensures that customer data remains secure and private.
- Cryptocurrency Payment Integration: Businesses can offer more tailored incentives and discounts for customers who use crypto payments, enhancing customer engagement.
"The seamless integration of blockchain data into e-commerce personalization strategies enhances customer satisfaction while providing the security and transparency needed for long-term loyalty."
Practical Steps for Implementing AutoML Solutions in Crypto-E-commerce
- Data Collection: Integrate blockchain transaction data, wallet history, and customer purchase behavior into a unified data lake.
- Model Training: Use AutoML platforms to train models on historical transaction data, focusing on identifying patterns in crypto-related purchases and token usage.
- Personalized Recommendations: Deploy the trained models to deliver product recommendations based on the customer's crypto profile and purchasing behavior.
Model Type | Use Case | Data Inputs |
---|---|---|
Classification | Predict likelihood of purchase | Transaction history, wallet type |
Clustering | Group users by crypto spending behavior | Spending patterns, token holdings |
Regression | Forecast future spending | Wallet size, previous purchases |
Automating Real-Time Decision-Making in Crypto Finance
The integration of automated machine learning (AutoML) into financial services is rapidly gaining momentum, especially in the highly volatile cryptocurrency market. The ability to analyze massive volumes of data in real-time and make accurate predictions has the potential to revolutionize trading strategies, risk management, and fraud detection. In cryptocurrency markets, where price fluctuations happen at lightning speed, decision-making systems must process and act on data almost instantaneously. AutoML allows for the creation of self-improving models that can continuously adapt to new patterns, enhancing decision-making accuracy over time.
As cryptocurrencies become more mainstream, the demand for advanced analytical tools grows. AutoML platforms are designed to streamline the data analysis process by automating model selection, hyperparameter tuning, and feature engineering. By reducing human intervention, businesses can focus on leveraging insights that drive faster and more informed decisions. Below are key benefits of integrating AutoML for real-time decision-making in the crypto-finance space:
- Instant Prediction Accuracy: AutoML enables systems to forecast price movements with greater precision, improving trading strategies and reducing the risks associated with volatility.
- Scalable Solutions: These platforms can scale efficiently to analyze large amounts of data from various crypto exchanges, social media sentiment, and market trends.
- Enhanced Risk Management: AutoML can detect unusual patterns, flagging potential risks or fraudulent activity before it impacts the market or investor portfolios.
Real-time decision-making is crucial for responding to market shifts as they occur. In this context, AutoML-driven systems provide a significant edge. Here's an overview of how it works:
- Data is collected from multiple sources, including blockchain transactions, exchange rates, and social media sentiment.
- AutoML models automatically preprocess the data, identifying the most relevant features and building predictive models.
- The system makes real-time predictions based on new data inputs, adjusting strategies and notifying stakeholders as needed.
"The cryptocurrency market operates 24/7, making it essential to leverage AutoML for fast, data-driven decision-making to stay ahead of market trends and mitigate risks."
In conclusion, the ability to automate decision-making processes in the fast-paced world of cryptocurrency is a game-changer for financial services. With real-time insights provided by AutoML platforms, companies can react to market fluctuations instantly and accurately, enhancing profitability and security.
Benefit | Description |
---|---|
Speed | Faster decision-making to match the speed of crypto markets |
Scalability | Handling large datasets from various crypto sources |
Risk Mitigation | Identifying threats or fraud in real-time |
Automated Audience Segmentation for Crypto Marketing Campaigns
In the rapidly evolving world of cryptocurrency marketing, precision is key to engaging the right audiences. Traditional methods of audience segmentation, such as demographic analysis or broad interest targeting, are becoming less effective in a market characterized by highly diverse and ever-changing user behavior. Automating this process using machine learning (ML) tools can dramatically enhance marketing strategies by allowing businesses to better understand and predict audience preferences, ultimately increasing conversion rates.
By leveraging automated solutions, crypto companies can segment their customer base dynamically, using real-time data and advanced algorithms to identify micro-segments within the broader audience. This provides marketers with insights that enable more tailored campaigns and content strategies, driving higher engagement in competitive markets. Here's how automating audience segmentation can improve marketing efforts in the crypto sector:
Key Benefits of Automating Audience Segmentation
- Enhanced Targeting: Machine learning models continuously analyze transactional and behavioral data, creating hyper-targeted groups based on real-time activity.
- Personalization: With deeper segmentation, marketers can deliver personalized offers and content to crypto users, improving the relevance of campaigns.
- Increased Efficiency: Automation reduces manual effort and ensures segmentation is always up-to-date, without the need for continuous human intervention.
Automating audience segmentation not only improves marketing accuracy but also drives ROI by targeting the right people at the right time.
Example Segments in Crypto Marketing Campaigns
Segment Type | Key Characteristics | Marketing Strategy |
---|---|---|
New Investors | Recently signed up, exploring cryptocurrency | Educational content, welcome bonuses, introductory webinars |
Active Traders | Frequent transactions, diverse portfolio | Advanced analytics, real-time alerts, exclusive market insights |
High Net-Worth Individuals | Large crypto holdings, high spending potential | Personalized investment advice, VIP access to new token launches |
With these insights, businesses in the crypto space can maximize their outreach by delivering content and offers that speak directly to each segment’s unique needs, ensuring better engagement and increased conversion rates in their marketing campaigns.
How Automl Improves Predictive Maintenance in Manufacturing
In the manufacturing industry, predictive maintenance is a critical component for optimizing production efficiency and reducing downtime. With advancements in artificial intelligence, the use of AutoML (Automated Machine Learning) has significantly enhanced the way predictive models are created and deployed. This technology allows businesses to automatically generate machine learning models, providing a more accurate understanding of equipment performance and potential failure points. By analyzing vast amounts of operational data, AutoML can predict when machines are likely to fail, enabling manufacturers to take proactive measures before problems arise.
Furthermore, AutoML is improving the scalability of predictive maintenance by simplifying the process of integrating machine learning into everyday operations. Traditionally, creating effective predictive models required specialized knowledge in data science, but AutoML democratizes this process, making it accessible even to non-experts. This not only reduces the time spent on model development but also enhances the overall efficiency and cost-effectiveness of the maintenance processes across the factory floor.
Key Benefits of AutoML for Predictive Maintenance
- Reduced Downtime: By predicting failures before they occur, AutoML enables maintenance teams to schedule repairs during non-peak hours, minimizing production losses.
- Cost Efficiency: Preventing unexpected breakdowns through early identification of potential issues reduces repair costs and extends the lifespan of machinery.
- Improved Accuracy: AutoML continuously refines models based on new data, leading to more precise predictions over time.
- Faster Implementation: The automation of model creation significantly reduces the time it takes to implement predictive maintenance strategies.
Steps in Integrating AutoML for Predictive Maintenance
- Data Collection: Gather sensor data and historical maintenance records from machinery.
- Data Preprocessing: Clean and prepare data for analysis, ensuring consistency and accuracy.
- Model Selection: AutoML algorithms automatically test various machine learning models to determine the best fit for the given dataset.
- Deployment: Implement the selected model into the production environment for real-time monitoring.
- Continuous Learning: The model is continuously retrained on new data to improve prediction accuracy.
Important: The use of AutoML in predictive maintenance not only boosts operational efficiency but also empowers businesses to become more proactive in managing machinery health.
Impact on Cryptocurrency Mining Industry
Factor | Impact of AutoML |
---|---|
Mining Equipment Maintenance | AutoML helps to predict failures in mining rigs, reducing downtime and ensuring consistent mining operations. |
Energy Efficiency | AutoML can optimize the maintenance schedule, reducing unnecessary energy consumption by ensuring equipment runs at peak performance. |
Cost Control | By predicting failures in advance, AutoML reduces the need for emergency repairs and extends the life of expensive mining hardware. |
Reducing Operational Costs with Automl in Supply Chain Management
The integration of Automl technologies within the supply chain has revolutionized how businesses manage their operations, especially in sectors like cryptocurrency and blockchain. By automating the model selection and tuning processes, companies can significantly reduce the overhead costs that traditionally come with supply chain optimization. Automl systems quickly analyze vast amounts of data, delivering efficient decision-making algorithms that improve logistics, inventory management, and demand forecasting. As a result, organizations can lower operational costs while increasing the overall accuracy of their forecasts.
In the context of cryptocurrency supply chains, Automl's ability to predict price fluctuations and optimize the allocation of resources can provide substantial cost reductions. Supply chains often face challenges such as inefficiencies, delays, and forecasting inaccuracies, which can be exacerbated by volatile market conditions. Automl offers a solution by automating predictive analytics, leading to optimized decisions in areas like procurement, transportation, and product delivery, all of which help cut operational costs.
Key Benefits of Automl in Cryptocurrency Supply Chains
- Cost Reduction: Automl systems automatically identify the most cost-effective strategies, optimizing inventory levels and reducing waste.
- Increased Efficiency: Automl streamlines decision-making, eliminating manual processes and reducing time spent on routine tasks.
- Improved Forecasting: Predictive models powered by Automl help forecast market trends and product demand, enabling better resource allocation.
Important: By automating supply chain operations, businesses in the cryptocurrency sector can minimize their exposure to price volatility and operational inefficiencies.
Operational Impact of Automl
- Reduced Lead Times: Automl helps streamline the entire supply chain, from procurement to final delivery, minimizing delays.
- Dynamic Risk Management: Automl tools enable real-time analysis, allowing businesses to quickly adapt to market changes and mitigate risks.
- Enhanced Resource Allocation: By predicting market shifts, Automl optimizes resource distribution, saving costs on excess inventory.
Cost Savings in Cryptocurrency Supply Chain
Supply Chain Activity | Traditional Cost | Automl-Optimized Cost |
---|---|---|
Inventory Management | High storage fees | Reduced excess inventory |
Logistics | High transportation costs | Optimized routes and timing |
Demand Forecasting | Inaccurate predictions | Better market insights and predictions |
Optimizing Cryptocurrency Models with Limited Data Using Automated Machine Learning
In the rapidly evolving world of cryptocurrency, building accurate prediction models with minimal historical data can be challenging. However, with the help of Automated Machine Learning (AutoML), it is possible to train and fine-tune machine learning models effectively even with small datasets. This approach leverages automated processes to select the most appropriate models, preprocess data, and tune hyperparameters, which significantly reduces the need for large-scale data and expert intervention.
In cryptocurrency trading, where data is often sparse or highly volatile, AutoML platforms can optimize models to predict price movements, identify trends, and detect potential anomalies in market behavior with only a fraction of the data. By automating the complex tasks of model training and fine-tuning, traders and analysts can focus on strategy development while relying on the robust predictive power of these AI-driven solutions.
Key Techniques for Efficient Model Development
AutoML platforms incorporate several techniques to improve model training and performance with minimal data:
- Data Augmentation: This method generates synthetic data from the available dataset, enabling models to learn more effectively from fewer real samples.
- Transfer Learning: Pretrained models can be fine-tuned on the available cryptocurrency data, accelerating the learning process without the need for large datasets.
- Hyperparameter Optimization: AutoML systems automatically adjust model parameters to find the optimal configuration for limited data scenarios.
Real-World Applications in Cryptocurrency
In cryptocurrency markets, AutoML can be used to:
- Price Prediction: Train models that predict cryptocurrency prices with minimal historical data by using advanced algorithms for trend analysis.
- Fraud Detection: Identify suspicious activities in blockchain transactions even when only a small sample of fraudulent cases is available.
- Risk Assessment: Automatically evaluate market risk by analyzing patterns in cryptocurrency data to improve investment decisions.
By leveraging AutoML, businesses can create highly accurate models even when faced with data limitations, leading to smarter, data-driven decisions in the cryptocurrency market.
Techniques | Benefits |
---|---|
Data Augmentation | Enhances model accuracy with limited data by generating synthetic samples. |
Transfer Learning | Speeds up model development by using pretrained models with small datasets. |
Hyperparameter Optimization | Fine-tunes model performance to achieve better results with minimal training data. |